A very popular tool for data scientists is RStudio. This tool allows you to interactively work with your R code, view the R console, the graphs and charts you create, manage the various objects and data frames you create, as well shaving easy access to the R help documentation. Basically it is a core everyday tool.
The typical approach is to have RStudio installed on your desktop or laptop. What this really means is that the data is pulled to your desktop or laptop and all analytics is performed there. In most cases this is fine but as your data volumes goes does does the limitations of using R on your local machine.
An alternative is to install a version called RStudio Server on an analytics server or on the database server. You can now use the computing capabilities of this server to overcome some of the limitations of using R or RStudio locally. Now you will use your web browser to access RStudio Server on your database server.
In this blog post I will walk you through how to install and get connected to RStudio Server on the Oracle BigDataLite VM.
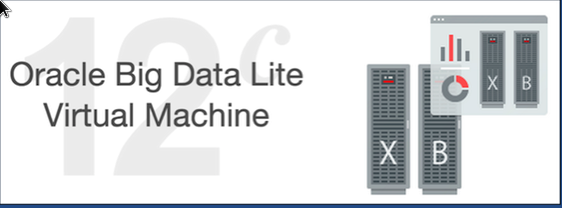
After starting up the Oracle BigDataLite VM and logging into the Oracle user (password=welcome1) you will see the Start Here icon on the desktop. You will need to double click on this.
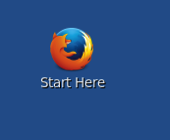
This will open a webpage on the VM that contains details of all the various tools that are installed on the VM or are ready for you to install and configure. This information contains all the http addresses and ports you need to access each of these tools via a web browser or some other way, along with the usernames and passwords you need to use them.
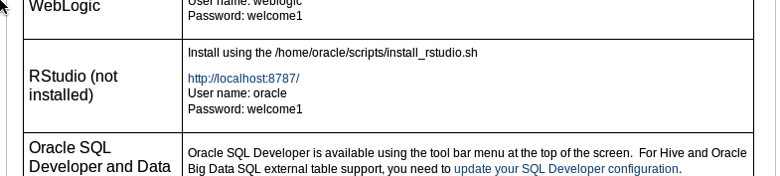
One of the tools lists is for RStudio Server. This product is not installed on the VM but Oracle has provided a script that you can run to perform the install in an automated way. This script is located in:
[oracle@bigdatalite ~]$ cd /home/oracle/scripts/
Use the following command to run the RStudio Server install script.
[oracle@bigdatalite scripts]$ ./install_rstudio.sh
The following is the output from running this script and it will be displayed in your terminal window. You can use this to monitor the progress of the installation.
Retrieving RStudio
--2016-03-12 02:06:15-- https://download2.rstudio.org/rstudio-server-rhel-0.99.489-x86_64.rpm
Resolving download2.rstudio.org... 54.192.28.12, 54.192.28.54, 54.192.28.98, ...
Connecting to download2.rstudio.org|54.192.28.12|:443... connected.
HTTP request sent, awaiting response... 200 OK
Length: 34993428 (33M) [application/x-redhat-package-manager]
Saving to: `rstudio-server-rhel-0.99.489-x86_64.rpm'
100%[======================================>] 34,993,428 5.24M/s in 10s
2016-03-12 02:06:26 (3.35 MB/s) - `rstudio-server-rhel-0.99.489-x86_64.rpm' saved [34993428/34993428]
Installing RStudio
Loaded plugins: refresh-packagekit, security, ulninfo
Setting up Install Process
Examining rstudio-server-rhel-0.99.489-x86_64.rpm: rstudio-server-0.99.489-1.x86_64
Marking rstudio-server-rhel-0.99.489-x86_64.rpm to be installed
public_ol6_UEKR3_latest | 1.2 kB 00:00
public_ol6_UEKR3_latest/primary | 22 MB 00:03
public_ol6_UEKR3_latest 568/568
public_ol6_latest | 1.4 kB 00:00
public_ol6_latest/primary | 55 MB 00:12
public_ol6_latest 33328/33328
Resolving Dependencies
--> Running transaction check
---> Package rstudio-server.x86_64 0:0.99.489-1 will be installed
--> Finished Dependency Resolution
Dependencies Resolved
================================================================================
Package Arch Version Repository Size
================================================================================
Installing:
rstudio-server x86_64 0.99.489-1 /rstudio-server-rhel-0.99.489-x86_64 251 M
Transaction Summary
================================================================================
Install 1 Package(s)
Total size: 251 M
Installed size: 251 M
Downloading Packages:
Running rpm_check_debug
Running Transaction Test
Transaction Test Succeeded
Running Transaction
Installing : rstudio-server-0.99.489-1.x86_64 1/1
useradd: user 'rstudio-server' already exists
groupadd: group 'rstudio-server' already exists
rsession: no process killed
rstudio-server start/running, process 5037
Verifying : rstudio-server-0.99.489-1.x86_64 1/1
Installed:
rstudio-server.x86_64 0:0.99.489-1
Complete!
Restarting RStudio
rstudio-server stop/waiting
rsession: no process killed
rstudio-server start/running, process 5066
When the installation is finished you are now ready to connect to the RStudio Server. So open your web browser and enter the following into the address bar.
http://localhost:8787/
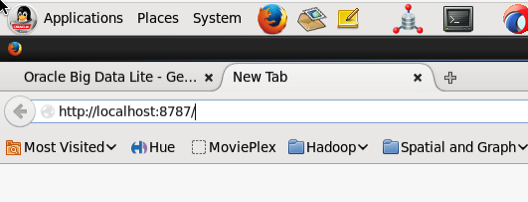
The initial screen you are presented with is a login screen. Enter your Linux username and password. In the case of the BigDataLite VM this will be oracle/welcome1.
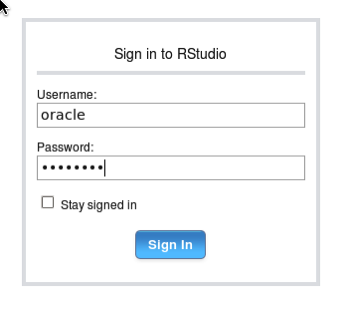
Then you will be presented with the RStudio Server application in your web browser, as shown below. As you can see it is very similar to using RStudio on your desktop. Happy Days! You are now setup and able to run RStudio on the database server.
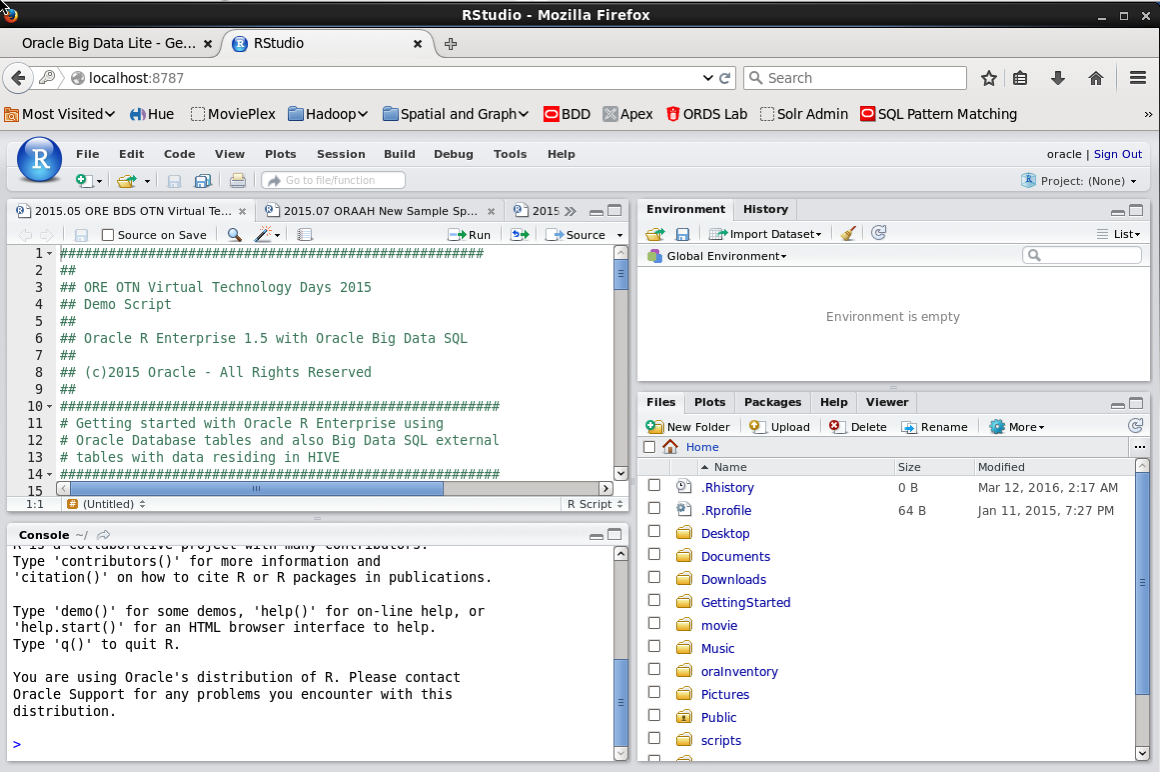
Make sure to log out of RStudio Server before closing down the window.
If you don't log out of RStudio Server then the next time you open RStudio Server your session will automatically open. Perhaps this is not the best for security, so try to remember to log out each time.
By now using RStudio Server on the Oracle Database server I can not get some of the benefits of computing capabilities of this server. Although there are still the typical limitations with of using R. But now I access RStudio on the database server and process the data on the database server, all from my local PC or laptop.
Everything is nicely setup and ready for you to install on the BigDataLite VM (thank you Oracle). But what about when we want to install RStudion Server on a different server. What are the steps necessary to install, configure and log in. Yes they should be similar but I will give a complete list of steps in my next blog post.