This is my second (of five) post on using Python to process Twitter data.
Check out my all the posts in the series.
In this post I was going to look at two particular aspects. The first is the converting of Tweets to Pandas. This will allow you to do additional analysis of tweets. The second part of this post looks at how to setup and process streaming of tweets. The first part was longer than expected so I'm going to hold the second part for a later post.
Step 6 - Convert Tweets to Pandas
In my previous blog post I show you how to connect and download tweets. Sometimes you may want to convert these tweets into a structured format to allow you to do further analysis. A very popular way of analysing data is to us Pandas. Using Pandas to store your data is like having data stored in a spreadsheet, with columns and rows. There are also lots of analytic functions available to use with Pandas.
In my previous blog post I showed how you could extract tweets using the Twitter API and to do selective pulls using the Tweepy Python library. Now that we have these tweet how do I go about converting them into Pandas for additional analysis? But before we do that we need to understand a bit more a bout the structure of the Tweet object that is returned by the Twitter API. We can examine the structure of the User object and the Tweet object using the following commands.
dir(user)
['__class__',
'__delattr__',
'__dict__',
'__dir__',
'__doc__',
'__eq__',
'__format__',
'__ge__',
'__getattribute__',
'__getstate__',
'__gt__',
'__hash__',
'__init__',
'__init_subclass__',
'__le__',
'__lt__',
'__module__',
'__ne__',
'__new__',
'__reduce__',
'__reduce_ex__',
'__repr__',
'__setattr__',
'__sizeof__',
'__str__',
'__subclasshook__',
'__weakref__',
'_api',
'_json',
'contributors_enabled',
'created_at',
'default_profile',
'default_profile_image',
'description',
'entities',
'favourites_count',
'follow',
'follow_request_sent',
'followers',
'followers_count',
'followers_ids',
'following',
'friends',
'friends_count',
'geo_enabled',
'has_extended_profile',
'id',
'id_str',
'is_translation_enabled',
'is_translator',
'lang',
'listed_count',
'lists',
'lists_memberships',
'lists_subscriptions',
'location',
'name',
'needs_phone_verification',
'notifications',
'parse',
'parse_list',
'profile_background_color',
'profile_background_image_url',
'profile_background_image_url_https',
'profile_background_tile',
'profile_banner_url',
'profile_image_url',
'profile_image_url_https',
'profile_link_color',
'profile_location',
'profile_sidebar_border_color',
'profile_sidebar_fill_color',
'profile_text_color',
'profile_use_background_image',
'protected',
'screen_name',
'status',
'statuses_count',
'suspended',
'time_zone',
'timeline',
'translator_type',
'unfollow',
'url',
'utc_offset',
'verified']
dir(tweets)
['__class__',
'__delattr__',
'__dict__',
'__dir__',
'__doc__',
'__eq__',
'__format__',
'__ge__',
'__getattribute__',
'__getstate__',
'__gt__',
'__hash__',
'__init__',
'__init_subclass__',
'__le__',
'__lt__',
'__module__',
'__ne__',
'__new__',
'__reduce__',
'__reduce_ex__',
'__repr__',
'__setattr__',
'__sizeof__',
'__str__',
'__subclasshook__',
'__weakref__',
'_api',
'_json',
'author',
'contributors',
'coordinates',
'created_at',
'destroy',
'entities',
'favorite',
'favorite_count',
'favorited',
'geo',
'id',
'id_str',
'in_reply_to_screen_name',
'in_reply_to_status_id',
'in_reply_to_status_id_str',
'in_reply_to_user_id',
'in_reply_to_user_id_str',
'is_quote_status',
'lang',
'parse',
'parse_list',
'place',
'retweet',
'retweet_count',
'retweeted',
'retweets',
'source',
'source_url',
'text',
'truncated',
'user']
We can see all this additional information to construct what data we really want to extract.
The following example illustrates the searching for tweets containing a certain word and then extracting a subset of the metadata associated with those tweets.
oracleace_tweets = tweepy.Cursor(api.search,q="oracleace").items()
tweets_data = []
for t in oracleace_tweets:
tweets_data.append((t.author.screen_name,
t.place,
t.lang,
t.created_at,
t.favorite_count,
t.retweet_count,
t.text.encode('utf8')))
We print the contents of the tweet_data object.
print(tweets_data)
[('jpraulji', None, 'en', datetime.datetime(2018, 5, 28, 13, 41, 59), 0, 5, 'RT @tanwanichandan: Hello Friends,\n\nODevC Yatra is schedule now for all seven location.\nThis time we have four parallel tracks i.e. Databas…'), ('opal_EPM', None, 'en', datetime.datetime(2018, 5, 28, 13, 15, 30), 0, 6, "RT @odtug: Oracle #ACE Director @CaryMillsap is presenting 2 #Kscope18 sessions you don't want to miss! \n- Hands-On Lab: How to Write Bette…"), ('msjsr', None, 'en', datetime.datetime(2018, 5, 28, 12, 32, 8), 0, 5, 'RT @tanwanichandan: Hello Friends,\n\nODevC Yatra is schedule now for all seven location.\nThis time we have four parallel tracks i.e. Databas…'), ('cmvithlani', None, 'en', datetime.datetime(2018, 5, 28, 12, 24, 10), 0, 5, 'RT @tanwanichandan: Hel ......
I've only shown a subset of the tweets_data above.
Now we want to convert the tweets_data object to a panda object. This is a relative trivial task but an important steps is to define the columns names otherwise you will end up with columns with labels 0,1,2,3...
import pandas as pd
tweets_pd = pd.DataFrame(tweets_data,
columns=['screen_name', 'place', 'lang', 'created_at', 'fav_count', 'retweet_count', 'text'])
Now we have a panda structure that we can use for additional analysis. This can be easily examined as follows.
tweets_pd
screen_name place lang created_at fav_count retweet_count text
0 jpraulji None en 2018-05-28 13:41:59 0 5 RT @tanwanichandan: Hello Friends,\n\nODevC Ya...
1 opal_EPM None en 2018-05-28 13:15:30 0 6 RT @odtug: Oracle #ACE Director @CaryMillsap i...
2 msjsr None en 2018-05-28 12:32:08 0 5 RT @tanwanichandan: Hello Friends,\n\nODevC Ya...
Now we can use all the analytic features of pandas to do some analytics. For example, in the following we do a could of the number of times a language has been used in our tweets data set/panda, and then plot it.
import matplotlib.pyplot as plt
tweets_by_lang = tweets_pd['lang'].value_counts()
print(tweets_by_lang)
lang_plot = tweets_by_lang.plot(kind='bar')
lang_plot.set_xlabel("Languages")
lang_plot.set_ylabel("Num. Tweets")
lang_plot.set_title("Language Frequency")
en 182
fr 7
es 2
ca 2
et 1
in 1
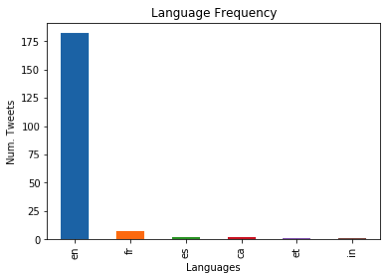
Similarly we can analyse the number of times a twitter screen name has been used, and limited to the 20 most commonly occurring screen names.
tweets_by_screen_name = tweets_pd['screen_name'].value_counts()
#print(tweets_by_screen_name)
top_twitter_screen_name = tweets_by_screen_name[:20]
print(top_twitter_screen_name)
name_plot = top_twitter_screen_name.plot(kind='bar')
name_plot.set_xlabel("Users")
name_plot.set_ylabel("Num. Tweets")
name_plot.set_title("Frequency Twitter users using oracleace")
oraesque 7
DBoriented 5
Addidici 5
odtug 5
RonEkins 5
opal_EPM 5
fritshoogland 4
svilmune 4
FranckPachot 4
hariprasathdba 3
oraclemagazine 3
ritan2000 3
yvrk1973 3
...
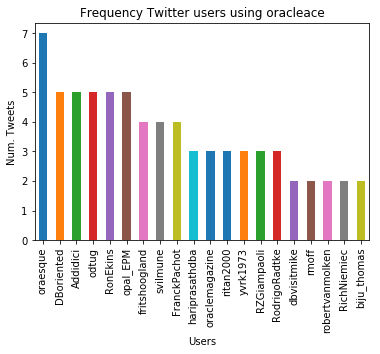
There you go, this post has shown you how to take twitter objects, convert them in pandas and then use the analytics features of pandas to aggregate the data and create some plots.
Check out the other blog posts in this series of Twitter Analytics using Python.